In ALLEGRO, we continue pushing the boundaries of machine learning (ML) models to enhance GSNR estimation and power optimization in optical networks. Our latest research evaluates key parameters influencing these models, including:
🔹 Channel activity & idle states across different frequency bands
🔹 Modulation format variations (max, min, avg, standard deviation)
🔹 Power levels at the span’s end
To achieve accurate predictions, we leveraged different ML models:
âš¡ Gradient Boosting (GB): Best accuracy but slower test-time execution
âš¡ Neural Networks (NN1 & NN2): Faster training with competitive results
âš¡ Power Estimation NN Models: Optimized for efficiency using Tanh & ReLu activation functions
💡 Key Takeaways:
✅ GB provides the most precise GSNR & power estimations, confirmed by KPIs such as MAE, MAPE, RMSE, and R²
✅ ML models can determine optimal power 25-50x faster than traditional analytical methods
✅ Power estimations across different models vary by only 0.1 dB, ensuring reliability
As AI continues to shape the future of optical networks, these findings highlight the balance between accuracy and computational efficiency in real-world deployments.
📢 How do you see ML transforming optical network optimization? Let’s discuss!
#OpticalNetworking #MachineLearning #GSNR #AI #ALLEGRO #Innovation
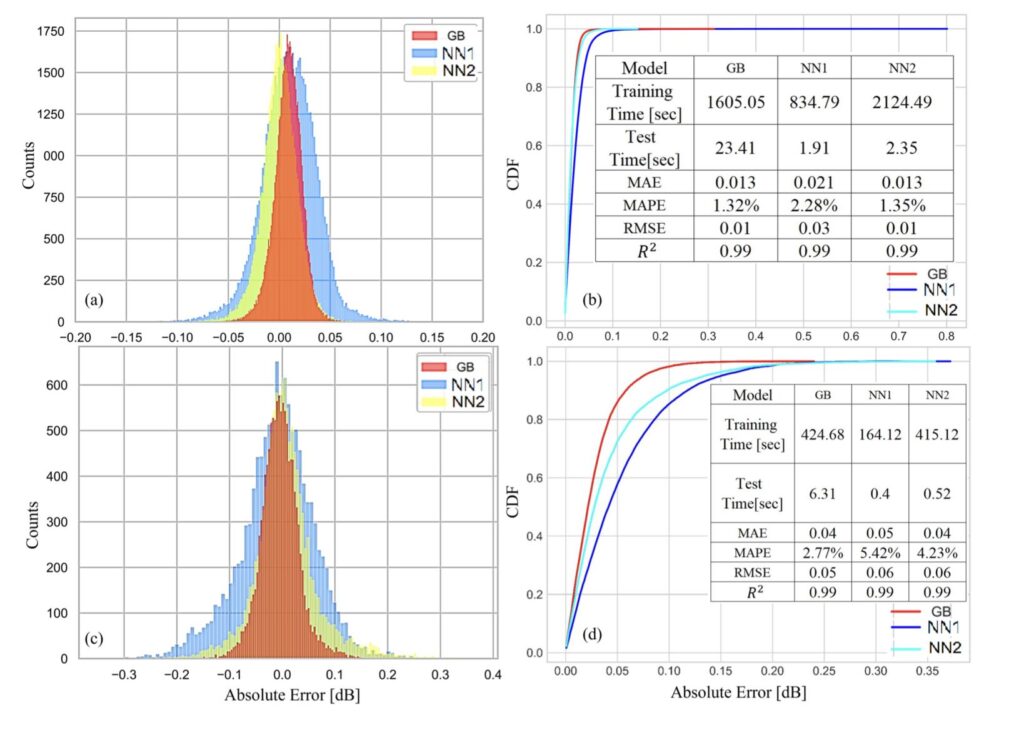