We’re excited to share a key innovation from our recent work in the ALLEGRO project β a complete ML pipeline for classifying impairments in Quantum Key Distribution (QKD) systems. βοΈπ‘
π While the QKD system is actively distributing keys, we collect sequential values of QBER (Quantum Bit Error Rate) and SKR (Secret Key Rate). These values form time series data which undergo the following pipeline:
1οΈβ£ Feature Extraction with tsfresh
β extracting rich time-series characteristics
2οΈβ£ Feature Selection using XGBoost
β reducing dimensionality while keeping the most impactful features
3οΈβ£ Classification via a deep neural network β with a 3-layer architecture (50x128x256x128x9) for robust state prediction
This end-to-end ML process enables real-time classification of network states, detecting various types of impairments without hardware dependencies β offering a powerful, technology-agnostic solution for the integration of QKD into classical networks.
π¬ This pipeline transforms raw metrics into actionable insights, enhancing the resilience and trustworthiness of quantum communications.
Stay tuned for more breakthroughs from the ALLEGRO team! π
#QuantumTech #MachineLearning #QKD #ALLEGROProject #Photonics #Cybersecurity #AIinTelecom #NeuralNetworks #TimeSeriesAnalysis #QuantumSecurity
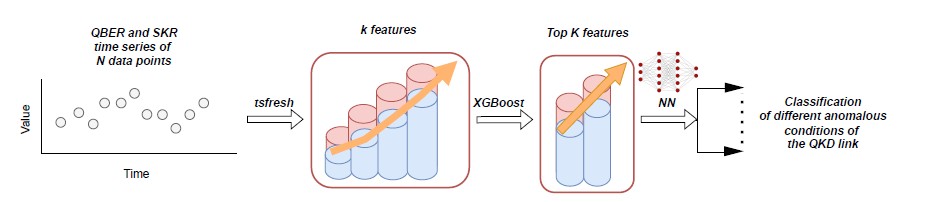